. Possibilities of neural network modeling in assessing of the sediments composition and properties relationship
Keywords:
sediments, particle size distribution, organic matter, neural network methodAbstract
На значительном фактическом материале продемонстрирована высокая эффективность нейросетевых методов при моделировании ряда параметров донных отложений. Используя многопараметрическую задачу нелинейной оптимизации, рассматриваемый подход позволяет прогнозировать изменчивость одних показателей состава и свойств донных отложений под воздействием других. Разработанный и апробированный в результате проведенного эксперимента алгоритм проектирования нейронных сетей с обратным распространением ошибки может быть использован для решения множества прикладных задач, возникающих при исследовании качества среды.
References
Бухарин С.В., Навоев В.В. Методы теории нейронных сетей в экспертизе технических и экономических объектов: монография. Воронеж: Научная книга, 2015. 274 с.
Дрейпер Н., Смит Г. Прикладной регрессионный анализ. Множественная регрессия. М.: Диалектика, 2007. 912 с.
Хайкин С. Нейронные сети: полный курс. М.: Вильямс, 2006. 1104 c.
Шерышева Н.Г., Ракитина Т.А., Поветкина Л.П. Условия формирования гранулометрического состава иловых отложений на территории национального парка «Самарская лука» // Самарская Лука: проблемы региональной и глобальной экологии. 2009. Т. 18, № 3. С. 104‒113.
Fausett L.V. Fundamentals of neural networks: architectures, algorithms and applications. Prentice-Hall, 1994. 461 p.
Hebb D.O. The Organization of Behavior: A neuropsychological theory. Lawrence Erlbaum Associates, 2002. 335 p.
Real-time: theory in practice // REX Workshop / Proceeding. Mook, The Netherlands, 1991. 723 p.
Jahanian F., Mok K. Safety analysis of timing properties in real-time systems /// IEEE Transactions of Timing Properties in a real-time systems. 1986. V. 12, № 9. P. 890‒904.
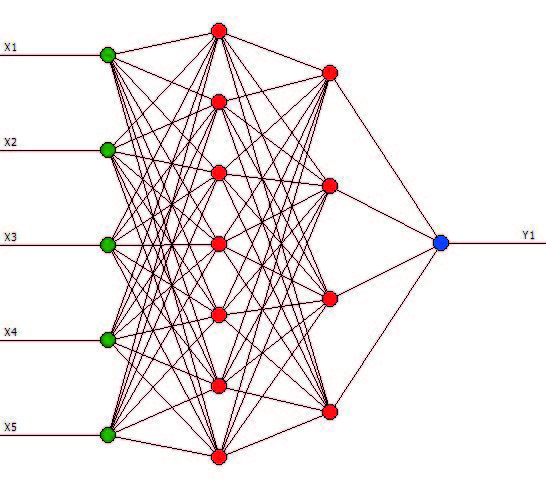